Key takeaways:
- Population genomics investigations usually analyse genomes in great quantities.
- Population genetics were able to connect the incompatible theories of Charles Darwin (natural selection) and Gregor Mendel (Mendelian inheritance).
- Human population genomics investigations mainly focus on diseases and healthcare: the major aim is to find genetic biomarkers for diagnostics and therapeutics.
- Microarrays and next-generation sequencing are the main technologies for population genetics and population genomics investigations.
What is population genomics
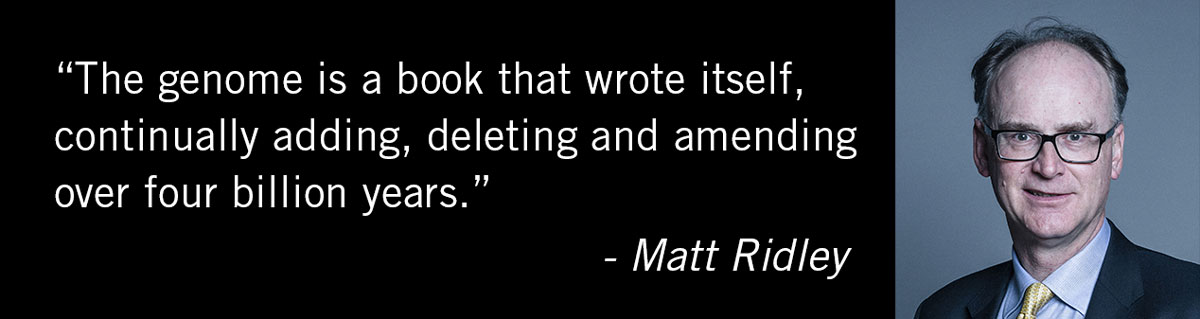
Population genomics is closely associated with population genetics, which aims to understand how various genetic traits are transferred from generation to generation.
Human population genetics focuses on the variations occurring within and between human populations due to various factors of evolution such as gene structures, chromosome positions and allele mutations.
Population genomics is more comprehensive and focuses on the entire human genome and the vast genome-wide association effects to identify genetic variations, the phylogenetic background (ancestry), demographics and microevolution in populations.
High-throughput sequencing (also referred to as next-generation sequencing (NGS )) and microarrays are the leading innovations that make it possible to compare genomes in great quantities and capture the diversity and variations of adaptations to a specific environment.
Population genetics and evolution: How it began
The foundation for population genetics and population genomics was laid by Friedrich Miescher in 1869 when he extracted DNA in the form of chromatin for the first time.
In 1902 and 1903, Theodor Boveri and Walter Sutton independently introduced the chromosome theory of inheritance. This theory stated chromosomes as structures containing genes at specific sites (loci) and that genes located on the same chromosome are passed on simultaneously when the entire chromosome is inherited (Boveri, 1902; Sutton, 1903).
Two other pioneers of population genetics and genomics are Alfred Wallace and Charles Darwin, who were the initial proponents of the evolution theory / theory of natural selection (image 1). They published their works in 1858/1859.
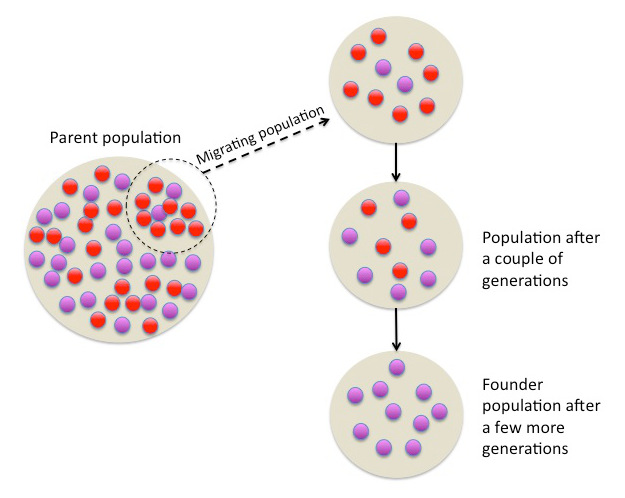
Image 1: Evolution can be observed in populations over time as parts of the population migrate. Specific genes start to change in their functions and/or frequencies, leading to the establishment of a founder population that shows reduced genetic variation.
Population genetics connected Charles Darwin’s theory of natural selection and Gregor Mendel’s laws of Mendelian inheritance.
Natural selection is the major driving force of evolutionary change. In a population, organisms possess various traits and tend to vary from each other. Organisms that are better adapted to their environment, based on their traits, are more likely to survive and reproduce than organisms that are less adapted. The advantageous traits are passed on to the offspring and, over time, these traits become more common.
Environmental pressures include food supply, predation and sexual selection.
Mendel’s theory is mainly based on his work with peas. He had two ‘pure breeding’ lines of pea:
1. Wrinkled seeds (WW)
2. Round seeds (RR)
Crossing of both lines results in an F1 generation with round seeds and no wrinkled seeds (WR).
Crossing of the F1 generation plants with each other results in F2 generation, where ¾ of plants have round seeds (RR, RW) and ¼ have wrinkled seeds (WW).
The results indicate that the R allele is dominant and its presence leads to round seeds (RR, WR). Only homozygous plants with the recessive W allele will have wrinkled seeds (WW).
This is called the law of segregation.
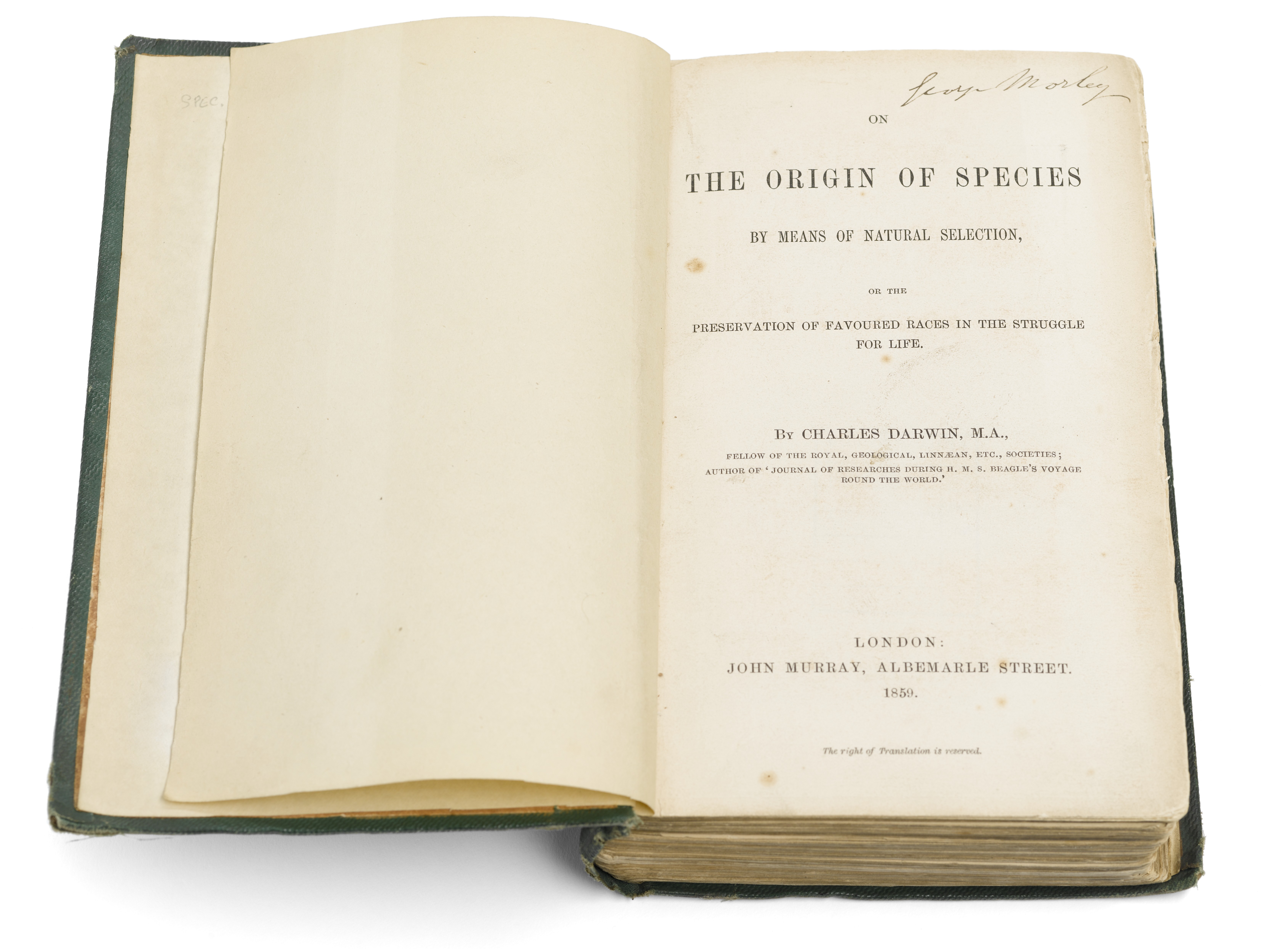
Image 2: 1859 edition of Charles Darwin’s The Origin of Species
The point that made both theories seemingly incompatible was the necessity of constant variation within a population for natural selection to change a population over time.
However, offspring tends to resemble their parents in traits (recessive and dominant alleles), i.e. traits are passed on to the next generation. So, where does the necessary variation for natural selection come from?
Researchers like Fisher, Wright and Haldane were able to gradually reconcile Mendel’s and Darwin’s theories and, thereby, established the disciplines of population genomics and genetics. They showed that a trait, which is affected by many alleles that each can lead to small differences in the trait, will be normally distributed in a population. A person’s height, for example, is mainly determined by the length of the bones in the legs, torso, neck and head. Variations in the length of the individual bones are influenced by many alleles, most with only a small effect on the overall height. Ronald A. Fisher showed that if, in this example, height is determined by many alleles, then the trait of height would be normally distributed in a population, i.e. there is a continuous range of variability. If natural selection (and sexual selection) favours increased height, the well-adapted individuals (survivors) would differ in mean height from the entire population. The offspring generation of the well-adapted individuals will differ from the preceding generation. If the underlying selection pressure continues, a big change in the mean height will eventually occur.
The mathematical models by Fisher, Wright and Haldane independently showed how evolutionary factors, such as natural selection and mutations, could alter the genetic composition of a population (Fisher, 1918; Haldane, 1930; Wright, 1931).
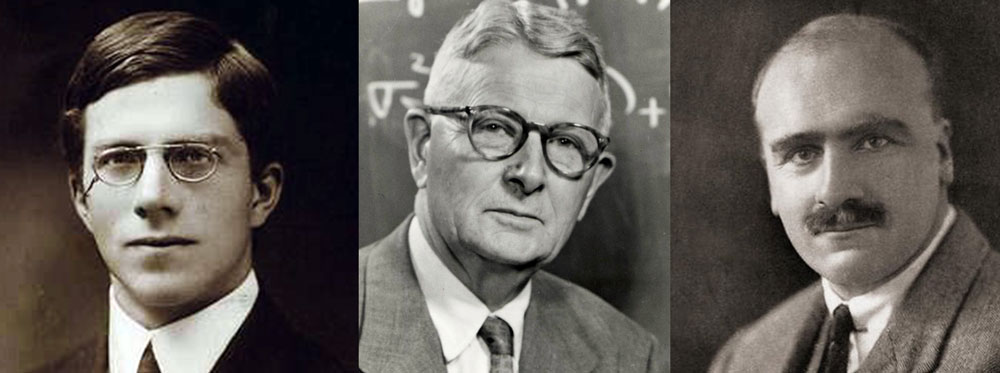
Image 3: From left to right: Ronald Aylmer Fisher, Sewall Wright and John Burdon Sanderson Haldane.
Population genetics in the present day
Population genomics investigations as they are done today were made possible by high-throughput sequencing and high-density microarrays. This gave researchers the possibility to access the entire genome of organisms.
Human population genomics mainly focuses on the topics of diseases and healthcare. Here, the genomes of thousands, if not millions, of humans are analysed in order to discover genetic biomarkers and therapeutic targets for diseases (Mattick, 2014).
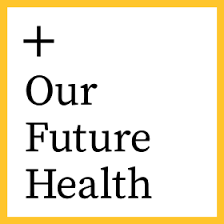
Large scale population genomics studies, such as the current Our Future Health study in the UK, with five million participants, aim to gain deeper insights and generate a comprehensive overview of the health of a population. The data will be used to advance the detection and treatment of diseases, and eventually improve disease prevention. Genetic biomarkers are of special significance for these endeavours. They are often categorised into biomarkers for diagnostics and biomarkers for drug response (therapeutic biomarkers).
Biomarkers for diagnostics are causative mutations for a disease, such as single nucleotide polymorphisms (SNPs) and insertions or deletions (InDels). The clinical test for lung cancer (bilateral stage IV well-differentiated adenocarcinoma) for instance, analyses the sequence encoding for an epidermal growth factor receptor (EGFR). A specific deletion on exon 19 of EGFR leads to increased cellular proliferation.
In this example of lung cancer, the deletion on exon 19 of the EGFR gene is also a biomarker for drug response (therapeutic biomarker). A specific therapeutic agent that inhibits EGFR function will result in tumour remission only if the deletion on exon 19 of EGFR has occurred. The same therapeutic agent will not necessarily be effective when a mutation on exon 20 of the EGFR gene, called T790M, is present. This deletion will also lead to increased cellular proliferation, however, as a different therapeutic biomarker that is responsive to a different therapeutic agent (Novelli et al, 2008; Templeton, 2018; Gambardella et al., 2020).
Population genomics is also used for the investigation of viral infections. Here, genetic variations and the evolution of genes in the viral genome lead to the emergence of different strains, such as the influenza viruses (Alpha-, Beta-, Gamma- and Deltainfluenzavirus) that emerge every year as new contagious strains (Image 4).
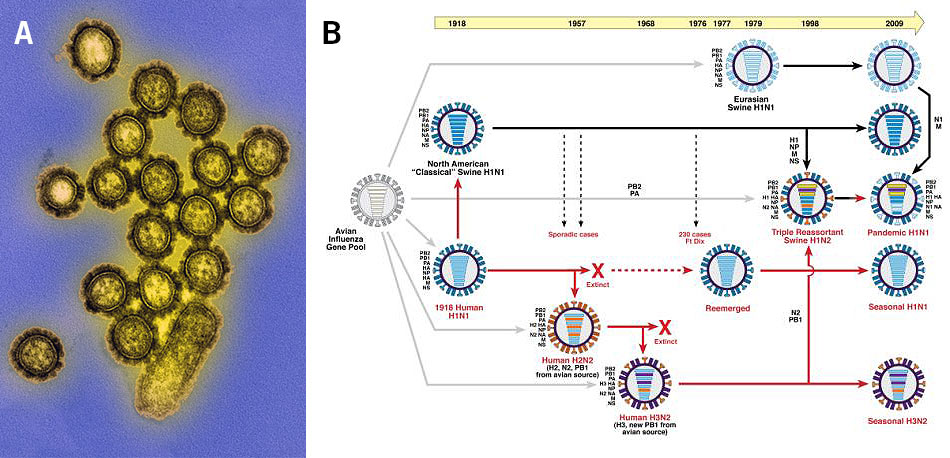
Image 4: Influenza viruses, a) transmission electron microscopy image of the H1N1 influenza virions (colourised), b) depiction of the genetic relationships between human and swine influenza viruses from 1918 until 2009 (red arrows: lineage of human influenza virus, black arrows: lineage of swine influenza virus, grey arrows: transfer of genes from the avian influenza A virus gene pool, horizontal bars in viruses: virus genes).
Microarrays and NGS for population genomics
Population genomics investigations require the sequencing or detection of a large amount of DNA from a large cohort (group of individual organisms that share a statistical factor like age).
Microarrays are often the go-to solution for these kind of investigations. Specifically, high-density microarrays are used to analyse the gene expression of an organism. They either cover the entire transcriptome or only specific genes of interest.
Quantifying the transcriptomes and analysing the differentially expressed genes of a large population could contribute to finding disease-related biomarkers.
High-density microarrays are available for humans/mammals, crops/plants, livestock and animals in aquaculture, including model organisms such as Arabidopsis thaliana and Drosophila melanogaster.
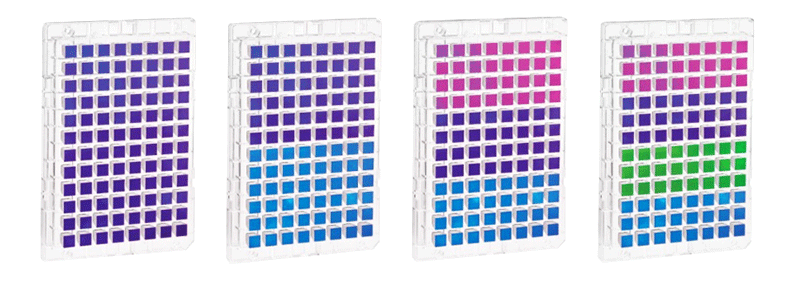
Image 5: High-density microarrays by Affymetrix.
The presence of SNPs within a population can also be investigated with microarrays. These SNP microarrays contain probes that are complementary to and bind specific gene sequences. However, the probes contain different SNPs and only bind gene sequences that also contain the respective SNPs. This way, the population can be screened for the presence of SNPs and, potentially, the effects of these SNPs can be inferred. Pre-designed microarrays tend to focus on more common gene variants, as the number of probes, and therefore SNPs, on a microarray is limited.
Nevertheless, microarrays are also used to analyse whether genomic amplifications, InDels or rearrangements have occurred or not.
Alternatively, gene expression and SNP analyses can be done with NGS. RNA sequencing (RNAseq) delivers unbiased data on differentially expressed genes, but is also used to analyse samples for splicing variants, gene isoforms, SNPs and even post-transcriptional modifications.
For NGS-based transcriptome sequencing, the sample RNA has to be converted into complementary DNA (cDNA) first. The cDNA is then used to prepare a library including ligation of adapters/UDIs to the cDNA fragments of the library. Following library prep, the cDNA fragments are sequenced and quantified in order to determine the differential gene expression levels.
The choice of a microarray-based or NGS-based analysis depends on the specifics and requirements of the research project. Both approaches have advantages and disadvantages and provide results that can differ to a degree.
A major advantage of microarrays is their long history of use. They are very well established and therefore, researchers are often more comfortable using them. Easy to use, consistently reliable results and regulatory approval are especially important for high-throughput applications and clinical settings. Also, it is still widely believed among scientists that microarrays are more cost-effective than NGS solutions. The cost-effectiveness of both technologies depends on the project requirements, research question and amount samples.
A disadvantage of microarrays is their reliance on probes and the data set the probes are based on. Microarrays can only return results for genomic regions that the probes have been designed for. Granted, data bases and sets are extensive and continuously updated.
NGS-based RNAseq does not rely on probes and provides a genome-wide analysis of the transcriptome without any knowledge of it. It also enables the analysis of novel transcripts and exon-exon junctions for example. However, the use of RNAseq requires the depletion of ribosomal RNA (rRNA) as it represents around 98% of the transcriptome. Only after rRNA depletion, can the RNA of interest be properly covered (Swindell et al., 2014; Rao, et al., 2019)
These points illustrate that the choice of microarray or NGS is quite complicated. Eurofins Genomics offers FREE consultation about what approach would suite your project the most and would be best to answer your scientific question. Get free consultation.
Or find out more about the Eurofins Genomics RNAseq solution INVIEW Transcriptome and our wide range of Illumina microarrays and Affymetrix microarrays.
By Aisha Maqsood and Dr Andreas Ebertz
Did you like this article about population genetics? Then subscribe to our Newsletter and we will keep you informed about our next blog posts. Subscribe to the Eurofins Genomics Newsletter.
One Comment